Last week, Lane Four had the privilege of attending and sponsoring Salesforce’s Agentforce World Tour Toronto—and what an incredible day it was! From lively conversations and super fun interactions at our booth, to an engaging theater session, the event was filled with opportunities to connect and share plenty of insights. In our session, “Key Lessons Learned for a Successful Agentforce Implementation,” Lane Four’s CEO, Andrew Sinclair, and Director of Architecture, Pete Gilbert, delivered valuable takeaways from our experience as one of Canada’s earliest adopters of Agentforce.
Agentforce’s AI-powered capabilities are proving to be instrumental in driving operational efficiency across the RevOps engine. However, like any industry-shaping tech, it comes with its own set of learning curves. In our presentation, we highlighted some of the must-know complexities and unique aspects of Agentforce implementation that we’ve encountered firsthand, showcasing how Lane Four continues to guide clients through these new challenges to unlock the platform’s full potential.
Whether you’re considering Agentforce or looking to optimize your current AI setup, these takeaways on data governance, credit consumption, testing, and managing your environment will guide you toward a smoother, more impactful journey. Let’s get to it!
Data Governance: The Foundation of AI Success
When it comes to Agentforce, data governance is non-negotiable. It’s the backbone of how AI agents operate, relying on structured, accessible, and well-categorized data to deliver accurate, context-driven responses. AI agents require access to data that is both comprehensive and appropriately governed to ensure optimal performance.
Despite its importance, many organizations grapple with defining a clear data governance strategy, often uncertain about their comfort levels when it comes to sharing data for AI-driven purposes.
To address this challenge, Lane Four developed a structured exercise to help clients clarify their approach by categorizing data according to risk levels. This method not only simplifies decision-making but also ensures that AI-driven solutions like Agentforce are built on a solid foundation of responsible data management.

The image above shows that data categories like public knowledge articles represent low-risk data, while sensitive customer information, like detailed purchase behaviours, carries higher risk. By understanding your organization’s risk tolerance, you can confidently prioritize use cases and scale AI solutions responsibly.

We’ve observed that early adopters often begin with use cases they can be less cautious about like public knowledge articles; gradually expanding to more ambitious AI applications as they gain confidence in the tool. Our advice? Take a gradual, phased approach—there’s no requirement to open the floodgates all at once to start leveraging AI. Start small, assess the ROI of initial implementations, and scale strategically. As trust in the technology grows, you can gradually expand to more complex or data-sensitive use cases, ensuring they align with your goals and drive meaningful impact.

Credit Consumption: Decoding the Complexities

When it comes to cost, Agentforce breaks away from traditional user-based licensing or subscription models. Instead, Salesforce has adopted a consumption-based pricing structure, which can initially feel a little bit confusing. Here’s what we’ve learned about managing credits effectively to optimize usage and maintaining control over costs:
- Agentforce Conversations
- The Most Expensive Credit
- Applied to conversations between agents and end customers.
- Covers all interactions within a single 24-hour messaging session unless ended by the agent or user.
- Data Cloud Credits
- The Most Abundant Credit
- Designed for a wide range of Data Cloud activities.
- The number of requests per prompt can vary significantly based on your specific use case.
- Metrics and feedback settings can significantly impact credit usage.
- Einstein Requests
- Fairly Abundant in Most Agreements We’ve Seen
- Used for all large language model (LLM) requests, both internal and external.
- Consumption depends on the API call size, with a multiplier applied accordingly.
- Like Data Cloud credits, the number of requests per prompt depends heavily on your use case.
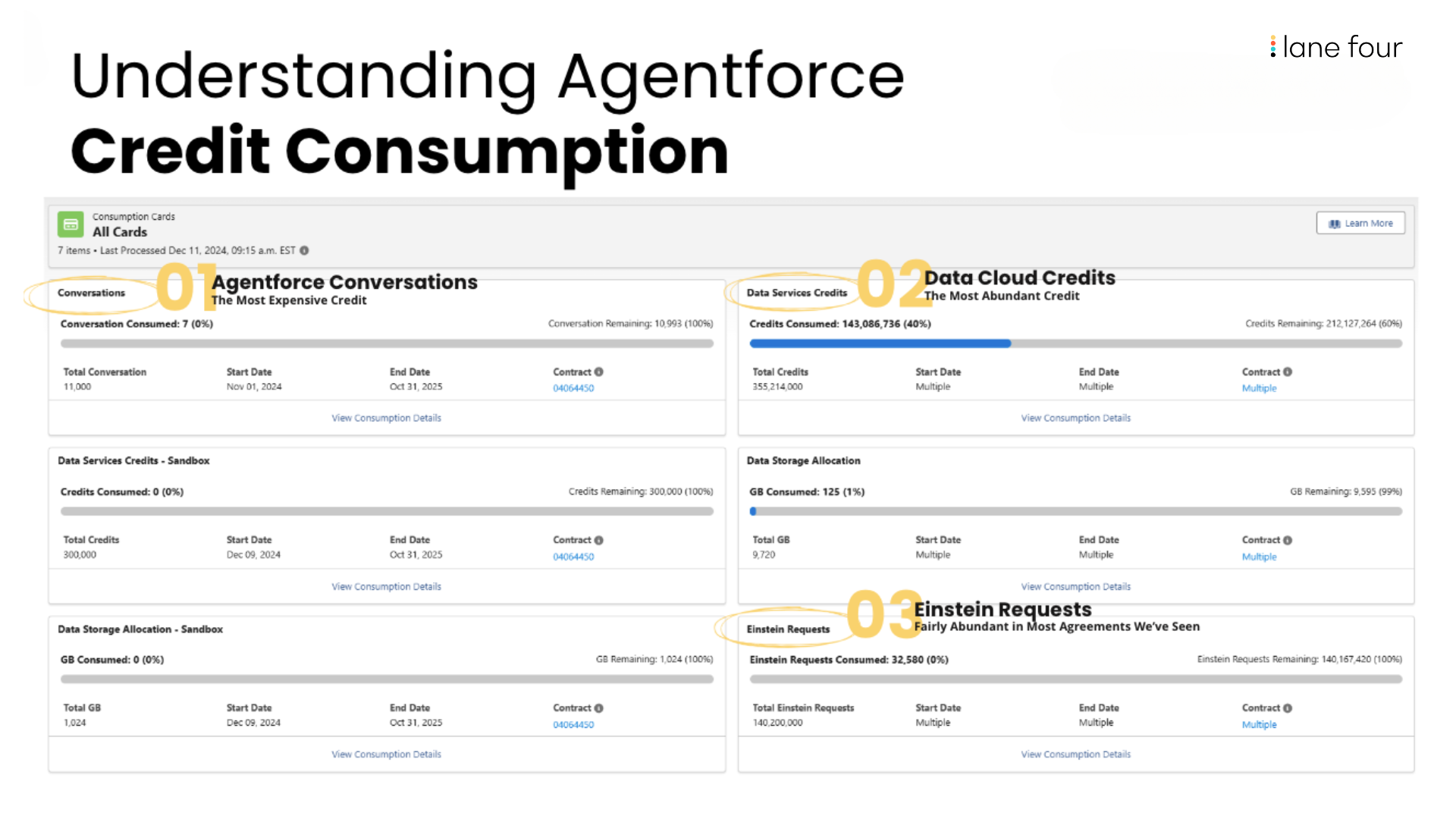
We recommend closely monitoring credit consumption during the first few weeks of implementation. Tracking trends, fine-tuning feedback settings, and targeting high-consumption areas will help you optimize and remain in the driver seat when it comes to usage and costs.
Quick Note: At the time of writing this presentation, the Agentforce Testing Centre had not yet been released, and details about its capabilities remain limited. However, we anticipate that this tool will significantly reshape the testing process, offering a more structured and robust approach. For clients with any degree of AI hesitancy, the Agentforce Testing Centre is likely to become an essential resource, providing critical insights and confidence in AI functionality.
Traditional Testing vs. Testing AI
Another critical aspect of an Agentforce implementation we needed to address is testing. Traditional testing methods simply don’t apply to AI solutions like Agentforce. Why? Because AI testing introduces unique challenges: outcomes depend on usage patterns, require high testing volumes, and demand a contextual understanding of prompts. Success isn’t as straightforward as a traditional pass/fail result—it’s measured as a percentage, reflecting overall performance and usage accuracy.
Traditional Testing
- Outcomes are typically clear-cut: pass or fail.
- Achieving test coverage across all scenarios is often feasible.
- Carefully designed test cases take priority over sheer volume.
- Success is measured by ensuring all unit tests pass.

AI Testing
- Response correctness can be subjective and context-dependent.
- Testing every possible use case or question is impractical.
- Performs better with high-volume testing rather than scenario-specific tests.
- Success is measured as a percentage, reflecting overall accuracy and performance.
We encourage taking a hands-on approach by exploring prompts and use cases directly in Copilot. This method not only fosters better engagement but also yields more reliable results. Testing for AI-driven solutions like Agentforce often consumes a much larger share of the implementation timeline compared to traditional tools. For instance, in one of our early Agentforce projects, the testing phase expanded from approximately 8% of the total cost to nearly 25%.
This increase is partly due to the blurred line between “testing” and “building” in AI projects. Unlike traditional tools, testing AI requires significant volume to ensure reliability, like we mentioned. Making UAT as frictionless as possible for testers is essential—especially with AI-driven tools. Encouraging employees to experiment with new topics and prompts in Copilot has proven far more effective than traditional UAT methods in a sandbox environment. This exploratory approach not only boosts engagement but also ensures your AI solutions are tested thoroughly in real-world scenarios.
Environment Management
Testing Agentforce requires Data Cloud-enabled environments, and Salesforce has introduced a significant improvement: Sandbox environments now share the same credit system as production. This alignment means greater feature parity, reducing surprises post-deployment.
We’ve also seen the value of activating tools like AI Audit Data and Prompt Builder Usage Metrics to track KPIs such as response satisfaction, engagement rates, and case deflection.
Post-Go-Live: Treat AI Like a Team Member!
AI agents require the same ongoing care as human agents. Regularly reviewing performance metrics and regression testing ensures they stay aligned with your evolving data and business needs.

Think of it like a performance review you’d hold with one of your human team members—what worked last quarter may need adjustments as your systems and customer behaviours change. Consistency in monitoring keeps your Agentforce solutions reliable and impactful.
Staying Agile in a Rapidly Changing Landscape
One of the biggest challenges of implementing transformative advancements in technology is keeping up with rapid updates. Some Salesforce resources released just months ago are already being flagged as outdated, and Agentforce 2.0 is already nearing! Staying informed and maintaining flexibility are critical for success especially when it comes to AI.
However, Lane Four’s expertise with Agentforce reflects our broader mission: continuing to help clients harness the power of tech that makes the most sense for them and their business/aligns with their business goals. Whether you want to move faster, or work more effectively, our presence at the Agentforce World Tour Toronto reinforced the importance of building strong relationships, both with clients and within the Salesforce ecosystem.
We’re excited to continue driving these AI advancements, sharing lessons learned, and supporting organizations on their journey toward AI readiness. Ready to explore what Agentforce can do for you? Let’s chat!